How Are AI Images Generated?
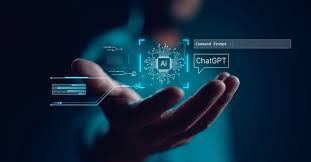
Artificial Intelligence (AI) has revolutionized the creation of images. AI’s ability to create realistic images has improved over time as a result of developments in neural networks and machine learning. We will explore the interesting field of AI image generation in this post and learn about the major methods used.
Understanding AI image generation
AI picture generation is the technique of producing images without the need for human input using neural networks and algorithms. Large image datasets are used to train these algorithms so that they can recognize patterns and features and generate new images that resemble the images present in the training set. Depending on the purpose of the AI model, the generated images can range from realistic photographs to artistic creations.
Main steps in AI image generation
1. Generative Adversarial Network (GAN): GAN revolutionizes the field of artificial intelligence picture creation. Generator and discriminator neural networks make up this system. The discriminator evaluates the hypothetical images created by the designer and provides comments on them. Through the competitive process, the designer optimizes his ability to create authentic visuals, resulting in exceptional results.
2. Variational Autoencoder, or VAE: Another popular technique for creating AI images. The neural network type known as VAE is capable of both encoding and decoding pictures. They work by processing the input image into an image by placing it in a small optical frame. By scanning for gaps in the image, the VAE is used to create new images.
3. Generative Adversarial Network (DCGAN) with Deep Convolution:** The GAN variant called DCGAN generates images using a convolutional neural network (CNN). CNNs can capture levels of features, which makes them ideal for image processing. DCGAN has produced high-quality images with excellent visual quality and has shown good results.
4. Transfer Method: Content-Preserving Version Transfer is the process of moving an image from one place to another. This technique is often used in artistic applications, allowing users to create unique and great visuals. To aid in creating visual content, AI algorithms are able to distinguish between the content and style of photos.
Challenges and future developments
Although much progress has been made in AI image generation, there are still many hurdles to overcome, including creating detailed photos, managing model outputs, and ensuring that a variety of images are created. To address these issues, researchers are putting a lot of effort into developing training techniques, datasets, and architectures. The future of AI imagery is bright, with applications across many industries, including entertainment, design, arts, and health. We should expect to see an even greater variety of images generated by AI systems as the models of these systems become more complex and data becomes more readily available. In summary, AI image generation is an exciting topic that allows cutting-edge technologies to create amazing and remarkable images. We can appreciate the complexity and inventiveness associated with creating AI graphics by understanding the steps and developments below.
Introduction:
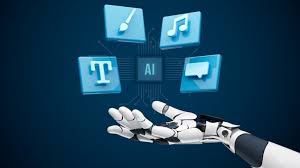
Artificial Intelligence (AI) has completely changed the way we interact with technology in the modern digital age. Creating visuals is an interesting use of AI. However, how accurate are these graphics created by AI? Let’s examine the interesting mechanisms behind artificial intelligence image production.
Understanding AI image generation:
AI imaging generation uses neural networks to create brand new, never-before-seen images. Large image datasets are used to train these neural networks, which generate new images based on the input data using learned features and models.
AI Image Processing Process:
Data collection: Collecting a large number of photos is the initial step in creating AI images. This dataset is the input used to train the neural network. Neural network training: Once the dataset is collected, methods such as variational autoencoders (VAE) and generative adversarial networks (GAN) are used to train the neural network on the data. During training the network learns to create images that are similar to real photos. Creating new images: The trained neural network can create new images by using random noise as input and transforming it into a meaningful image. This process involves multiple levels of networks, which combine them to create complex layouts and designs.
Challenges in the AI ​​Imaging Generation:
Despite its progress, AI image generation still has problems. Creating realistic, high-quality images that are hard to distinguish from real photos is one of the biggest hurdles. Since the neural network copies patterns from the training data in the images it creates, another problem is to ensure that the diversity of the images it creates is maintained.
Applications of AI image generation:
Different industries can use AI technology in different ways. For example, AI can be used to create original and cutting-edge artwork in the field of design and art. Images generated by AI can help with medical diagnosis and treatment in the healthcare industry. AI is able to create characters and environments in games. When it comes to AI generating tools, the possibilities are almost limitless.
Conclusion:
In conclusion, AI image generation is an interesting field that is constantly evolving and pushing the boundaries of technology. AI is capable of creating amazing and inventive graphics with the use of neural networks and sophisticated algorithms. The potential of AI image generation is endless as we continue to investigate its capabilities.